Edge AI: The Future of Embedded Intelligence
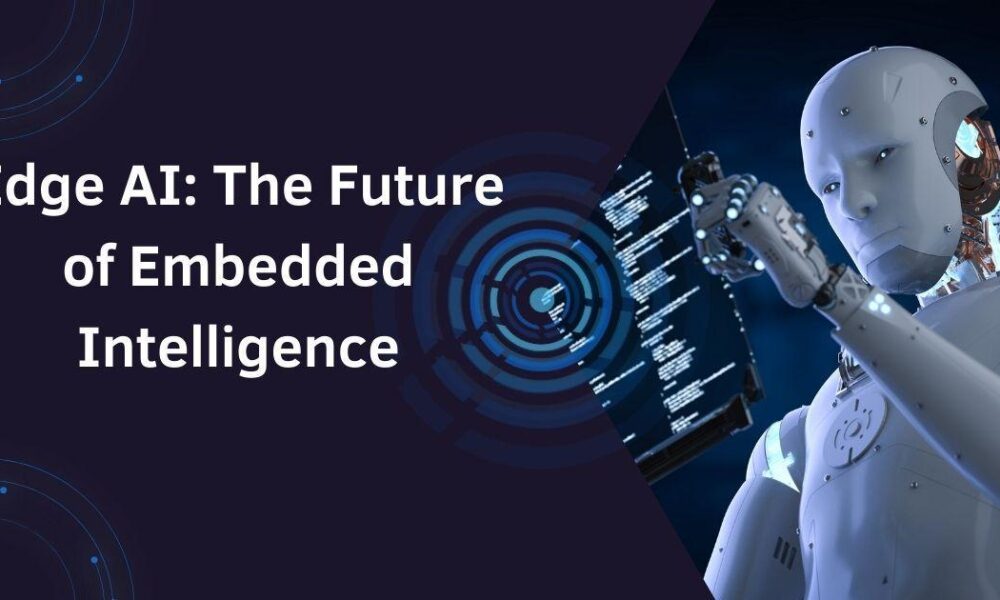
In today’s digital era, artificial intelligence is rapidly evolving, reshaping the way we process and analyze data. Moving away from cloud-reliant models, Edge AI is revolutionizing embedded systems by enabling real-time, on-device processing. This paradigm shift is significantly reducing latency, lowering operational costs, and strengthening security by keeping data closer to its source. Anushree Nagvekar, an expert in the field, delves into the groundbreaking innovations driving this transformation and their profound implications for the future of computing.
A Shift from Cloud to On-Device Processing
Traditional AI processing depended on cloud computing, resulting in high latency, increased costs, and security risks. Edge AI overcomes these challenges by executing computations directly on embedded hardware, reducing processing times to just 3–15 milliseconds, compared to the 150–1200 milliseconds seen in cloud-based AI. This shift enhances responsiveness and efficiency while minimizing reliance on external servers. Additionally, Edge AI drives significant cost savings, with organizations reporting a 72% reduction in data transmission costs and a 51% drop in system maintenance expenses. By lowering cloud dependency, businesses are achieving substantial operational efficiencies, making Edge AI a compelling solution for modern computing needs.
Hardware Innovations Powering Edge AI
Edge AI leverages specialized hardware accelerators like NPUs and advanced memory architectures for efficiency and performance. Modern NPUs, featuring systolic arrays, execute up to 8,192 MAC operations per cycle while maintaining thermal efficiency within a 5W power envelope. Memory advancements include hierarchical designs with 2MB–8MB on-chip SRAM and LPDDR5 interfaces, reducing access latencies by 65%. Smart caching algorithms further enhance efficiency, achieving over 92% hit rates for deep learning workloads, making Edge AI both powerful and energy-efficient.
Security and Privacy Advantages
Edge AI enhances security by processing data locally, reducing cyber threat exposure and shrinking the attack surface by 85% compared to cloud systems. Organizations report a 63% drop in security incidents, while industries like healthcare and finance see data breaches decrease by 82.5%. Keeping sensitive data on-site minimizes unauthorized access risks and simplifies regulatory compliance. This localized approach strengthens data privacy, making Edge AI essential for secure, efficient, and compliant computing across various sectors.
Enhancing Performance in Autonomous Systems
Edge AI is revolutionizing real-time decision-making in autonomous systems by enabling self-driving vehicles to process sensor data locally, achieving rapid response times of just 8.5 milliseconds—far faster than cloud-based alternatives. By integrating high-resolution cameras, LiDAR, and radar, Edge AI cuts decision latency by over 90%, significantly enhancing safety, reliability, and efficiency. This advancement ensures more responsive and intelligent autonomous operations across various applications.
Optimized Power Management for Efficiency
With increasing demands on embedded systems, power efficiency is a crucial factor. Edge AI systems now employ dynamic voltage and frequency scaling (DVFS), allowing devices to adjust power consumption based on workload demands. Recent implementations have demonstrated a 52.3% reduction in power usage while maintaining peak performance.
Intelligent workload management further enhances power efficiency. Edge AI processors now anticipate computational requirements with 94% accuracy, enabling proactive power adjustments. This has resulted in devices achieving extended operational runtimes while reducing energy costs by up to 56.7%.
The Road Ahead for Edge AI
The future of Edge AI promises significant advancements, with next-generation NPUs expected to offer up to 12.5× the performance of current models while cutting power consumption by 78.3%. AI accelerators may soon exceed 80 TOPS/W, enabling highly complex computations with minimal energy use. Additionally, hybrid AI architectures are emerging, dynamically balancing edge and cloud processing to enhance efficiency. These systems aim to reduce latency by 82.5% and infrastructure costs by 42.3%. As machine learning-driven orchestration evolves, Edge AI will become more adaptive, seamlessly integrating into a wide range of applications.
In conclusion, Edge AI is redefining embedded computing by making artificial intelligence more efficient, secure, and cost-effective. By shifting from cloud-dependent processing to on-device intelligence, Edge AI is unlocking new possibilities across industries. With continuous innovations in hardware, security, and power management, the future of AI is undoubtedly at the edge. As Anushree Nagvekar highlights, these advancements are not just improving existing systems—they are shaping the next era of intelligent computing.